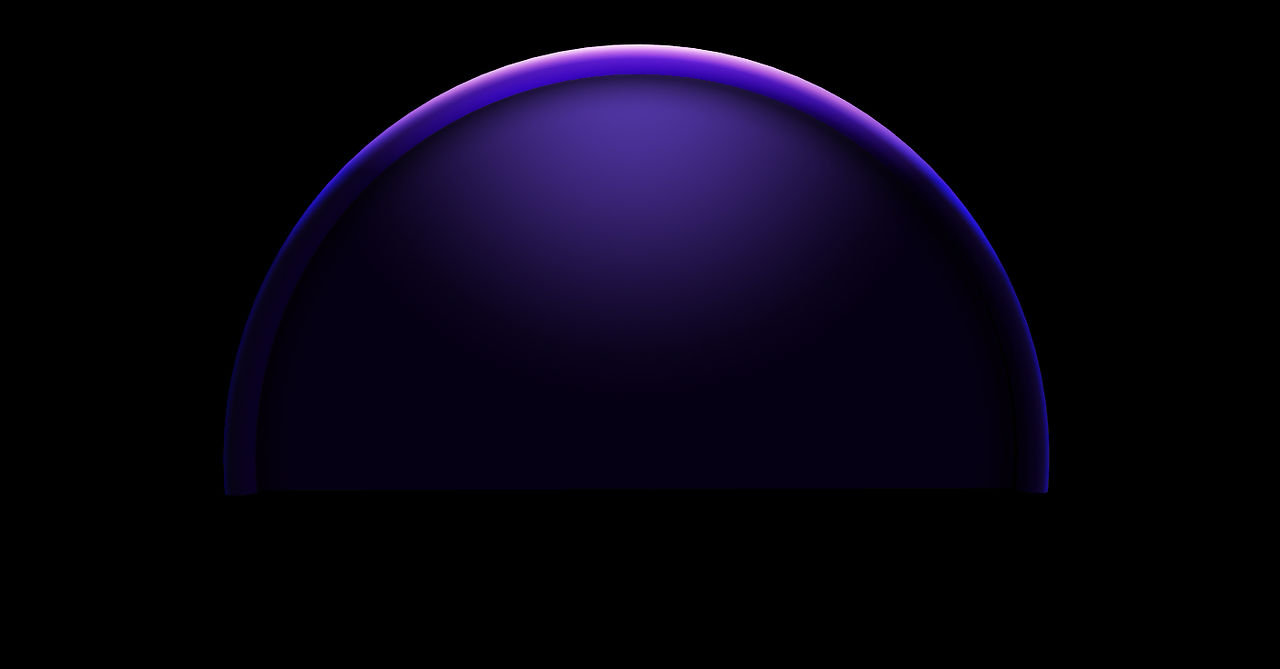

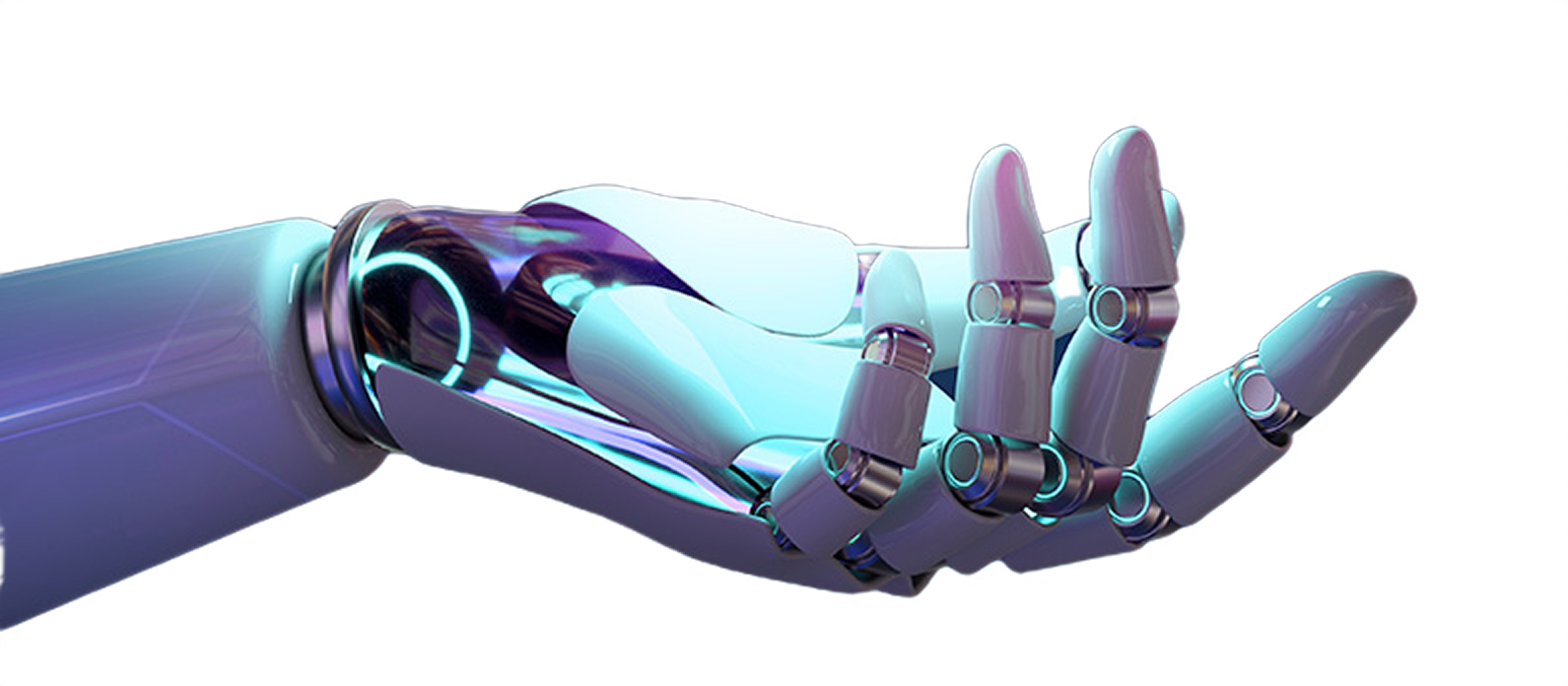
The Cybersapient Advantage: Precision & Partnership
Dedicated Teams & Talents
We're not just service providers; we're your strategic technology partners. At Cybersapient, we understand that every business is unique. Our team of seasoned AI engineers, cloud architects, and cybersecurity experts collaborate closely with you to design and implement solutions that align with your specific goals. We prioritize transparency, communication, and delivering measurable results
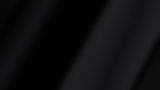
“ The project has been in capable hands, and the team has demonstrated exceptional responsiveness, engagement, and a genuine commitment to making the project the best possible. ”
Al Ameen R
Designation - co founder, paisa on click
![IMG-20241125-WA0049[1].jpg](https://static.wixstatic.com/media/ae0b1c_f79aac8b31f642858532b7d3e499df6d~mv2.jpg)
Deep Dive into Our Expertise
Interactive Element: Interactive service cards with expandable sections, including case studies and technical specifications.
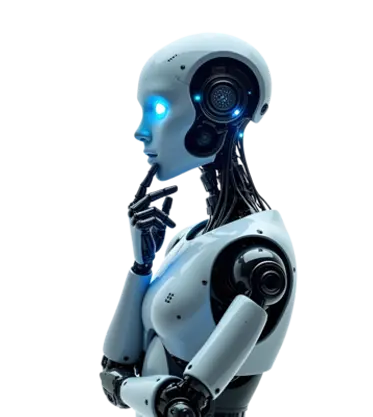
Our Specialized Services: Engineered for Peak Performance.

AI-Driven Product Engineering
Service Highlights
We leverage deep learning, computer vision, and natural language processing to build intelligent products that automate decision-making and enhance user experiences. We specialize in developing AI-powered recommendation systems, predictive analytics tools, and smart automation platforms.
"We helped a retail client develop an AI-driven inventory management system that reduced stockouts by 25% and improved forecasting accuracy by 30%."

Agent Development
Create sophisticated, autonomous agents for customer service, data analysis, and process automation. We build agents that learn, adapt, and interact naturally with users.
"We developed a virtual assistant for a healthcare provider that handles patient inquiries, schedules appointments, and provides personalized health information."

LLM Integration (Open Source & Public)
Details: Integrate state-of-the-art large language models like GPT-3, BERT, and open-source alternatives into your applications. We specialize in natural language understanding, text generation, and conversational AI.
"We integrated a fine-tuned LLM into a content creation platform, enabling users to generate high-quality marketing copy and technical documentation."

Generative UI
Design user interfaces that adapt dynamically to user behavior and preferences. We use generative AI to create personalized and intuitive user experiences.
"We built a generative UI for an e-commerce platform that dynamically adjusts product recommendations and layout based on user browsing history."

MLOps | AIOps | DevOps
Implement robust MLOps, AIOps, and DevOps pipelines to streamline the development, deployment, and management of your AI and cloud applications. We ensure scalability, reliability, and security.
"We automated the deployment of machine learning models for a financial services company, reducing deployment time by 50% and improving model performance by 15%."
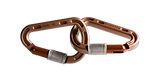
Cybersecurity - OffenseLogic
Proactive threat detection and response with our OffenseLogic methodology. We simulate real-world attacks to identify vulnerabilities and strengthen your security posture.
“We performed penetration testing and vulnerability assessments for a government agency, identifying and mitigating critical security flaws.”

Multi-Cloud and Hybrid IT Infrastructure
Design and manage scalable and secure multi-cloud and hybrid IT infrastructures. We specialize in AWS, Azure, and Google Cloud Platform, ensuring seamless integration and optimal performance.
"We migrated a large enterprise's legacy infrastructure to a hybrid cloud environment, reducing operational costs by 20% and improving system reliability."
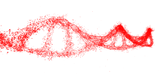
SaaS Development
Build robust and scalable SaaS applications with microservices architecture and cloud-native technologies. We focus on performance, security, and user experience.
"We developed a SaaS platform for a logistics company that manages delivery routes, optimizes resource allocation, and provides real-time tracking."
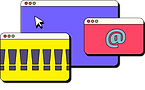
Mobile Development
Develop native and cross-platform mobile applications for iOS and Android. We specialize in building user-friendly and high-performance mobile experiences.
"We created a mobile application for a fitness company that tracks workouts, provides personalized training plans, and integrates with wearable devices."

AI-Powered Automation & RPA
Automate repetitive tasks and streamline workflows with AI-powered RPA solutions. We use machine learning to automate complex processes and improve efficiency.
“We implemented RPA to automate invoice processing for a manufacturing company, reducing processing time by 60% and minimizing errors.”
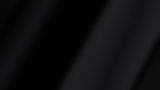

Need tech talents?
Need a team with expertise? We are here to help, talk to our TA partners. Our expert team will help you to build and scale your team that aligns with your business needs.
Building a product?
Do you have a product idea and want to build it? Talk to our product managers, who have delivered hundreds of functional products. Don’t worry about the tech and process; we have a battle-tested process in place.
Talk tech?
If you want to have a simple tech chat with someone who speaks your language, we are here for you.
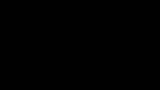

Blogs
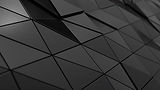
-
We will respond to you within 24 hours.
-
We’ll sign an NDA if requested.
-
You'll be talking to product and tech experts (no account managers).
AI-Powered Automation & RPA
We're ready to discuss your specific needs and challenges. Schedule a free consultation with our experts to explore how Cybersapient can help you achieve your business goals.
Schedule Your Free Discovery Session
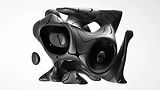