AI and Big Data: How it is transforming Nutrition Research
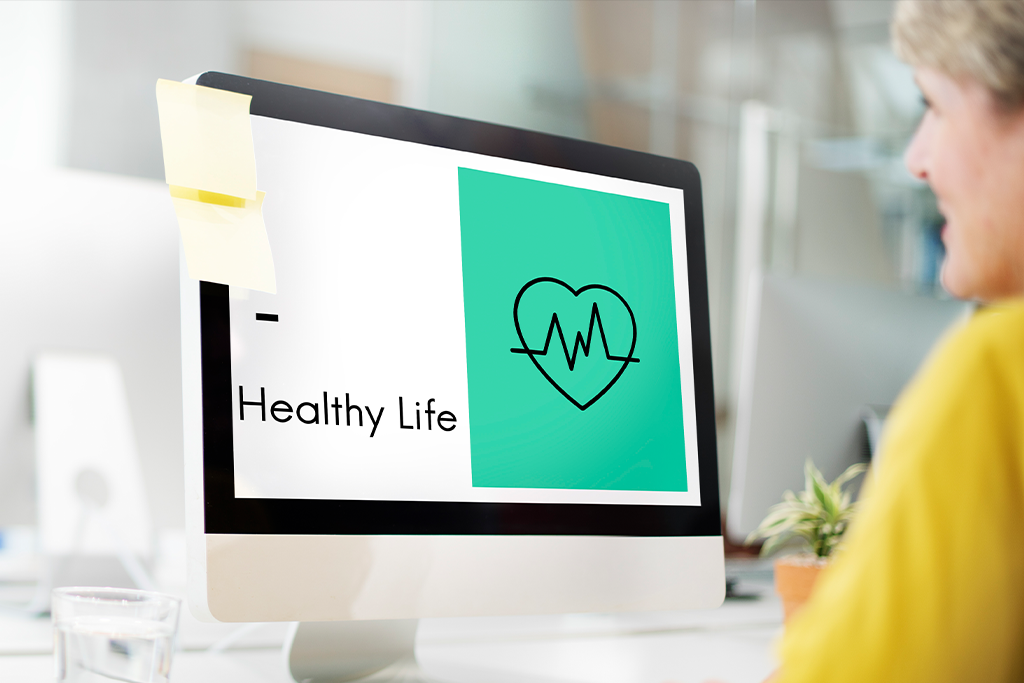
AI and Big Data in Nutrition Research
AI and Big Data have enormous potential to transform the field of nutrition research by allowing for the efficient processing and analysis of large amounts of dietary data. Machine learning algorithms can be used to identify patterns and relationships in complex nutrition datasets, leading to better insights into the links between diet and health. The use of AI and Big Data can ultimately lead to more personalized and evidence-based nutritional recommendations for individuals and populations.
Use of Machine Learning Algorithms in Analyzing Nutrition Data
Machine learning algorithms can be used to analyze large amounts of nutrition data, including dietary intake and biomarker data. These algorithms can identify patterns and relationships that are not immediately apparent to humans, allowing for more precise and accurate assessments of nutrient intake and dietary quality. The use of machine learning in nutrition research has the potential to improve our understanding of the complex interactions between diet and health and inform more effective interventions to improve health outcomes.
Big Data Analytics in Dietary Assessment and Nutritional Epidemiology
Big data analytics can help improve the accuracy and efficiency of dietary assessments and nutritional epidemiology studies by enabling the analysis of large datasets. These analyses can identify dietary patterns and trends, and help identify factors that contribute to variations in nutrient intake and health outcomes. The use of big data analytics in nutrition research has the potential to generate important insights into the relationships between diet and health, and inform policies and interventions aimed at improving population health.
The Role of AI and Big Data in Identifying Nutrition-Related Health Risks
AI and Big Data can be used to identify individuals and populations at higher risk of nutrition-related health conditions, such as obesity, diabetes, and heart disease. By analyzing large amounts of health and dietary data, machine learning algorithms can identify patterns and risk factors that are associated with these conditions, allowing for earlier interventions and more effective prevention efforts. The use of AI and Big Data in identifying nutrition-related health risks has the potential to improve population health outcomes and reduce healthcare costs associated with these conditions.
Nutrient Profiling and Food Labeling: How AI is Improving Accuracy
AI can improve the accuracy of nutrient profiling and food labeling by analyzing large amounts of food composition data and identifying patterns and relationships. This can lead to more accurate nutrient content claims and health claims on food labels, as well as improved dietary guidance and recommendations for consumers. The use of AI in nutrient profiling and food labeling has the potential to improve public health outcomes by promoting healthier food choices and reducing the risk of diet-related chronic diseases.
The Use of AI and Big Data in Clinical Nutrition Trials
AI and Big Data can be used to improve the design and analysis of clinical nutrition trials. Machine learning algorithms can help identify patient subgroups that may benefit more from specific nutritional interventions, allowing for more personalized treatment approaches. Additionally, the use of AI and Big Data can help optimize trial design, reduce costs, and improve the efficiency of clinical nutrition research.
Ethical Considerations in the Use of AI and Big Data in Nutrition Research
The use of AI and Big Data in nutrition research raises important ethical considerations around issues such as data privacy, data ownership, and potential biases in algorithmic decision-making. It is important to ensure that the collection, use, and analysis of nutrition data are done ethically and transparently, with appropriate safeguards in place to protect individual privacy and ensure the responsible use of data. Furthermore, it is critical to address any potential biases that may arise in algorithmic decision-making to ensure that nutritional recommendations are evidence-based and equitable
Future Directions in AI and Big Data for Nutrition Research
The future of AI and Big Data in nutrition research holds immense promise for improving our understanding of the complex relationships between diet and health. Areas of future research include the development of more sophisticated machine learning algorithms that can analyze multiple sources of nutrition data, the integration of AI and Big Data into clinical practice and the development of more personalized nutrition recommendations based on individual factors such as genetics, microbiome, and lifestyle. Additionally, there is potential for the use of AI and Big Data in the development of novel interventions and technologies to improve nutrition-related health outcomes.
The Promising Future of AI and Big Data in Advancing Nutrition Science.
The future of AI and Big Data in nutrition science is full of promise. The ability to analyze large amounts of dietary data using machine learning algorithms has the potential to transform our understanding of the relationships between diet and health, leading to more effective prevention and treatment of nutrition-related health conditions. Ultimately, the use of AI and Big Data in nutrition science has the potential to improve population health outcomes and reduce healthcare costs associated with diet-related chronic diseases.
1 reply.
user
August 25, 2023
test