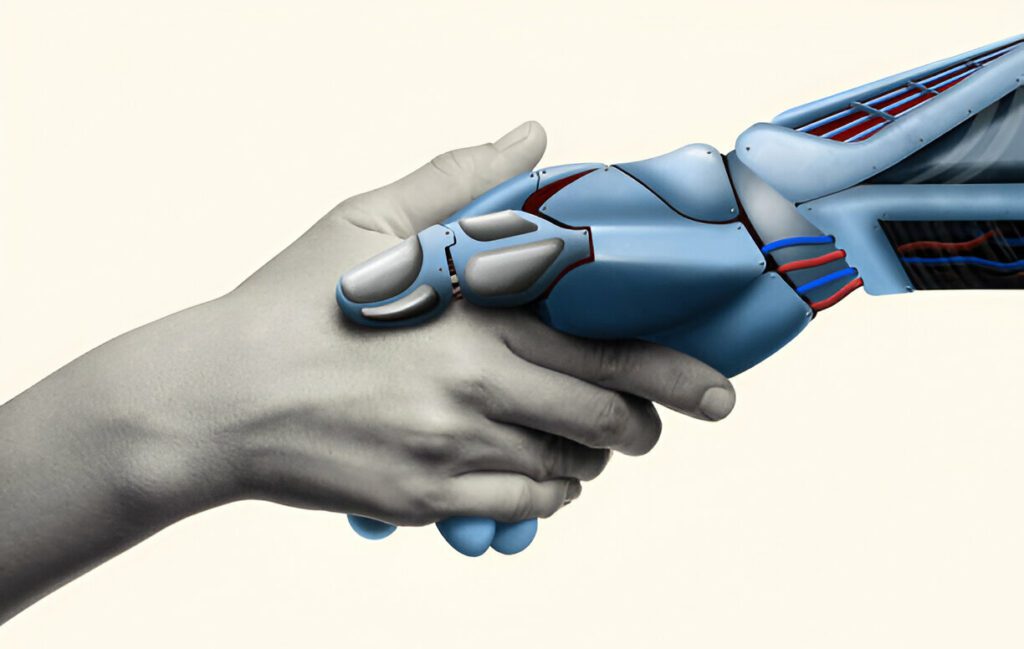
The adoption of Artificial Intelligence (AI) is surging. Companies are eager to leverage AI’s capabilities to streamline operations and unlock new possibilities. A recent Slack survey underlines this trend, revealing a 24% rise in AI usage within workplaces, with 96% of executives believing AI integration is crucial for their success.
However, despite this enthusiasm, a significant trust gap persists. Only 7% of desk workers trust AI outputs for work-related tasks. This disparity highlights the disconnect between executive optimism and employee skepticism. Several key concerns fuel this apprehension:
Understanding the Concerns:
- Bias and Fairness: AI systems can perpetuate existing societal biases, leading to discriminatory outcomes. Organizations must strive to build unbiased AI models by employing diverse datasets and auditing for potential fairness issues.
- Privacy and Security: Employees worry about their data being collected, stored, and utilized by AI systems. Implementing robust data privacy measures and adhering to regulations like GDPR are essential steps in building trust.
- Black Box Decision-Making: The inner workings of many AI systems remain opaque, making it difficult to understand how they arrive at decisions. Explainable AI (XAI) techniques can help demystify the decision-making process and foster trust.
- Automation Anxiety: There’s widespread fear that AI will automate jobs, leading to unemployment. The focus should shift towards human-AI collaboration, leveraging AI’s power to enhance human capabilities and drive innovation.
Building a Foundation of Trust:
Legislation and ethical frameworks are playing a crucial role in addressing trust concerns. Regulatory bodies are moving forward with initiatives like:
- Data Protection and Privacy Laws: Regulations like GDPR establish a framework for responsible data collection, storage, and usage. This ensures that AI systems handle personal data with utmost care and respect.
- AI Regulations: The EU’s AI Act aims to regulate AI based on risk levels. High-risk AI systems will require stricter controls around data quality, documentation, transparency, and human oversight. This promotes transparency and accountability throughout the AI lifecycle.
Standards for Trustworthy AI:
Beyond legislation, industry standards empower organizations to implement responsible AI practices. Initiatives like:
- NIST AI Risk Management Framework: This framework guides organizations in managing AI-related risks, including assessing context, quantifying risks, and implementing mitigation strategies.
- Generative AI Profile: Recognizing the potential risks posed by generative AI models, NIST has published a profile addressing concerns around disinformation and promoting ethical applications.
- ISO/IEC 23894 Standard: This comprehensive standard offers a systematic approach to identifying and managing AI risks throughout its development, deployment, and decommissioning.
Earning Public Trust in the Long Term:
Building enduring public trust in AI requires a multi-pronged approach:
- Comprehensive Risk Assessment: Organizations need to conduct thorough assessments to identify potential ethical and compliance issues associated with their AI systems.
- Cross-Functional Teams: Establishing teams with representatives from legal, compliance, IT, and data science ensures all aspects of AI development and deployment are considered.
- Robust Governance: Implementing strong governance structures with clear policies and procedures guarantees transparency and accountability in AI initiatives.
- Regular Audits and Monitoring: Regularly auditing AI systems for bias and inaccuracies, and utilizing monitoring tools to track performance and adherence to regulations, is crucial.
- Employee Education: Ongoing training sessions on AI ethics, regulations, and best practices ensure employees feel informed and engaged with AI integration.
- Detailed Record-Keeping: Maintaining meticulous records of AI development processes, data usage, and decision-making criteria allows organizations to provide reports to regulators if needed.
- Engagement with Regulators: Building relationships with regulatory bodies allows organizations to stay abreast of developing regulations and contribute to shaping future policies.
Contextualizing AI for Trust:
For trustworthy AI, data integrity is paramount. Generative AI’s reliance on large datasets doesn’t necessarily equate to accuracy. Retrieval Augmented Generation (RAG) offers a solution. RAG combines static large language models with context-specific data, enhancing accuracy by retrieving relevant information from a comprehensive knowledge base.
Benefits of RAG: RAG empowers organizations to build purpose-driven AI applications that are highly accurate, context-aware, and adaptable. This can lead to improved decision-making, enhanced customer experiences, and greater operational efficiency.
Conclusion:
Bridging the AI trust gap requires a commitment to transparency, accountability, and ethical AI development. Businesses have an array of tools and strategies at their disposal to achieve this. Implementing robust data privacy measures, adhering to regulations, continuously auditing AI systems, and openly communicating with stakeholders are all critical steps. Building trustworthy AI is an ongoing endeavor, but it’s an essential one, paving the way for a future where humans and AI can collaborate effectively for a better tomorrow.