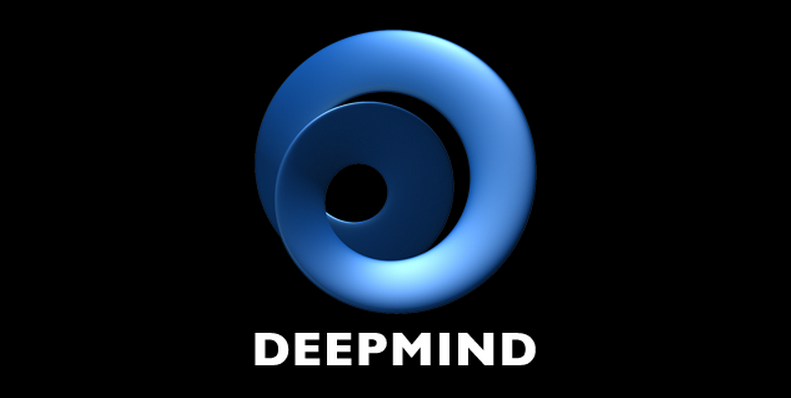
Introduction
The landscape of generative AI is rapidly advancing, influencing sectors such as medicine, education, finance, art, and sports. These strides are largely due to AI’s enhanced ability to learn from vast datasets and construct intricate models with billions of parameters. Despite the significant scientific discoveries, new business opportunities, and industrial growth fueled by these advancements, the financial and environmental costs of training these expansive models are substantial. The computational power needed for generative AI models results in high energy consumption and a notable carbon footprint.
Historically, efforts to make generative AI more sustainable have focused on improving hardware efficiency and developing smaller models with fewer parameters. However, Google DeepMind has introduced a novel approach with their JEST (Joint Example Selection) algorithm, which trains models 13 times faster and is ten times more power-efficient than existing methods.
In this article, we will explore the challenges associated with AI training and how the JEST algorithm addresses these issues. Additionally, we will examine the broader implications and future directions for JEST, envisioning its potential impact on AI training’s speed, cost-efficiency, and environmental sustainability.
Challenges of AI Training: High Costs and Environmental Impact
Financial Costs
Training generative AI models is an expensive endeavor. For instance, training OpenAI’s GPT-3, which has 175 billion parameters, costs around $4.6 million. Training ChatGPT-4 is estimated to have cost OpenAI approximately $100 million. These expenses are primarily due to the enormous computational resources, extensive data processing, and prolonged training times required.
Energy Consumption
Generative AI training processes are extremely energy-intensive, involving thousands of GPUs and consuming multiple gigawatt-hours of energy. Data centers that house the computing infrastructure for AI training consume about 200 terawatt-hours (TWh) of electricity annually, roughly 1% of global electricity demand. A McKinsey report predicts that data center power consumption in the US could rise from 17 gigawatts (GW) in 2017 to 35 GW by 2030, necessitating the output equivalent of nine Hoover Dams to meet this additional demand.
Carbon Footprint
The high energy consumption associated with training generative AI models significantly contributes to greenhouse gas emissions, exacerbating climate change. A study by the University of Massachusetts Amherst found that training a large AI model can emit as much carbon dioxide as five cars over their lifetimes. Specifically, training a single AI model can emit more than 626,000 pounds of CO2, equivalent to the carbon footprint of 315 trans-American flights.
These challenges primarily stem from the reliance on high-energy-consuming computational hardware and the inefficiency of current training algorithms. While the AI community has made strides in developing energy-efficient hardware, there is a pressing need for smarter algorithms that optimize data usage and reduce training times. Google’s JEST algorithm is pioneering this research, enhancing the efficiency of AI training by intelligently selecting the most valuable data.
Understanding the JEST Algorithm
JEST is designed to train multimodal generative AI models more efficiently. To understand its workings, consider AI training as solving a complex puzzle, where each piece (data point) contributes to the complete picture (AI model). JEST acts like an experienced puzzle solver, making the process more efficient by selecting the most important and distinctive pieces.
JEST employs a smaller AI model to evaluate the quality of data batches, ranking them based on their effectiveness in model training. By prioritizing and selecting the most informative batches, JEST speeds up the training process significantly.
A key aspect of JEST’s approach is multimodal contrastive learning, which focuses on understanding the correspondence between different data types, such as text and images. This method helps JEST evaluate the effectiveness of multimodal data samples in training the model. By assessing the collective learnability of data samples, JEST selects a small, informative batch from a larger “super batch,” ensuring rich learning opportunities and efficient training.
Looking Forward: JEST Beyond Faster, Cheaper, Greener AI Training
The implications of JEST extend beyond mere efficiency and cost savings. Here’s how JEST can continue to transform the field of generative AI:
Enhanced Model Performance and Accuracy
JEST’s innovative data selection approach leads to faster training times and improved model performance. By focusing on the most informative data batches, JEST ensures AI models are trained on high-quality inputs, enhancing their accuracy and robustness.
Identifying and Mitigating Bias in Data
AI is often prone to biases due to unbalanced datasets. JEST’s data selection process prioritizes diverse and representative samples, helping reduce biases. For example, in healthcare AI applications, JEST can select data encompassing various demographic factors, ensuring that medical diagnostic models are trained on a diverse patient population.
Facilitating Innovation and Research
By reducing the computational resources and time required for AI training, JEST lowers the barriers to entry for researchers and innovators. This accessibility fosters a vibrant AI development ecosystem, enabling smaller teams and organizations to experiment with and deploy advanced AI solutions.
Promoting Inclusive AI Development
Inclusive AI development involves diverse perspectives to effectively address biases and ethical concerns. JEST’s ability to select data based on its informational value encourages inclusive practices in dataset curation. By involving multidisciplinary teams in defining data selection criteria, AI developers can ensure JEST effectively addresses biases and ethical considerations.
The Bottom Line
DeepMind’s introduction of the JEST algorithm marks a significant leap forward in generative AI training. By significantly speeding up training processes and reducing energy consumption, JEST offers substantial cost savings and addresses environmental concerns linked to AI development. Beyond these advantages, JEST has the potential to enhance model accuracy, mitigate data biases, foster innovation, and promote inclusive AI development. As JEST continues to evolve, it promises to redefine the future of AI, advancing towards more efficient, sustainable, and ethically responsible AI solutions.