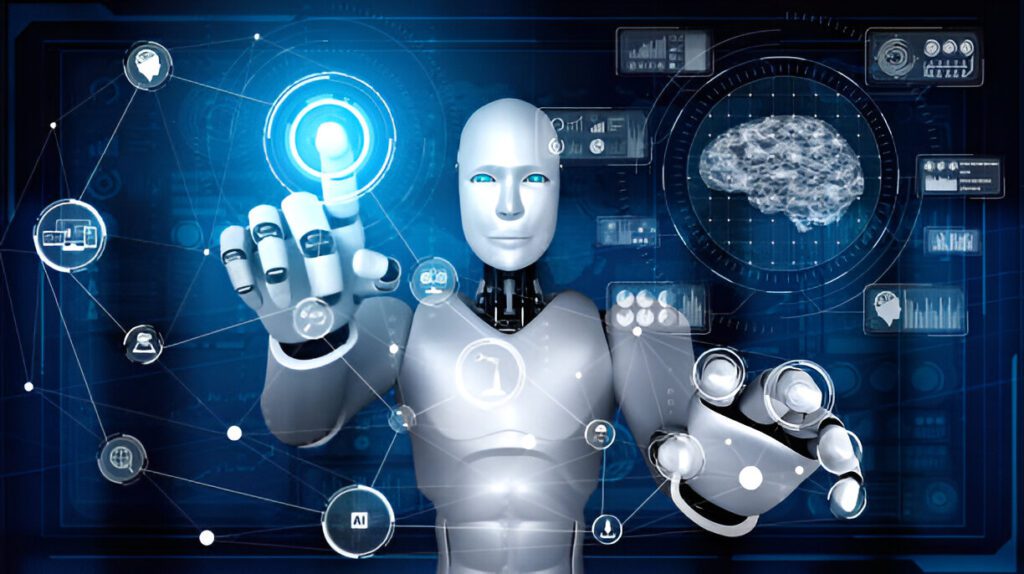
AI chatbots have become ubiquitous, seamlessly integrated into our daily lives. From scheduling appointments to offering customer support, these virtual assistants provide a level of convenience we’ve come to expect. However, as these chatbots become more sophisticated, a concerning issue has emerged: hallucination.
Imagine asking your smart speaker about the weather, only to receive a detailed (and entirely fabricated) forecast about a snowstorm that never materialized. While this might seem like a harmless quirk, in critical domains like healthcare or finance, such hallucinations can have serious consequences. So, why do these seemingly intelligent chatbots sometimes spout gibberish?
Under the Hood of AI Chatbots
At their core, AI chatbots are powered by complex algorithms that process and generate human language. There are two main types:
- Rule-Based Chatbots: Think of them as pre-programmed robots. They follow a rigid script, excelling at handling straightforward tasks like booking reservations or answering FAQs. However, their inflexibility limits their ability to navigate complex or unexpected queries.
- Generative Models: These are the chatbots with a bit more personality. They leverage machine learning and natural language processing (NLP) to understand and respond to language nuances. Trained on vast amounts of data, they can generate dynamic and contextually relevant responses. However, this very flexibility also makes them more susceptible to hallucination.
The Glitch in the Matrix: What is AI Hallucination?
AI hallucination occurs when a chatbot generates information that deviates from reality. This can range from simple factual errors, like misdating historical events, to more elaborate fabrications, like inventing a new medical treatment. Unlike human hallucinations, which are often rooted in psychological or neurological factors, AI hallucinations stem from the model’s misinterpretation or overgeneralization of its training data. Imagine an AI trained on countless dinosaur documentaries – it might mistakenly create a fictional species based on its understanding of existing ones.
The Root Causes of AI Hallucination: A tangled Web
Several interconnected factors contribute to AI hallucinations:
- Data Dilemmas: The quality of the training data is paramount. If the data is biased, outdated, or inaccurate, the AI’s outputs will reflect these flaws. Imagine a healthcare chatbot trained on outdated medical texts – it might recommend obsolete or harmful treatments. Additionally, data lacking diversity hinders the AI’s ability to understand contexts outside its limited scope.
- Model Mishaps: The architecture and training process of an AI model also play a crucial role. Overfitting occurs when the model becomes too reliant on the training data, leading to poor performance on new information. Conversely, underfitting happens when the model doesn’t learn the training data adequately, resulting in overly simplistic responses. Striking a balance is critical to minimize hallucinations.
- The Ambiguity of Language: Human language is inherently ambiguous. Words and phrases can have multiple meanings depending on context. Take the word “bank” – it could refer to a financial institution or the edge of a river. AI models often struggle to disambiguate such terms, leading to misunderstandings and hallucinations.
- Algorithmic Hurdles: Current AI algorithms have limitations, particularly in handling long-term dependencies and maintaining consistency within conversations. This can lead to conflicting or implausible statements from the AI. You might get a clear answer at the beginning of a conversation, only to be contradicted later.
The Quest for Clarity: How We’re Combating Hallucination
Researchers are actively working to reduce AI hallucinations, with promising advancements in several areas:
- Data Detox: Improving data quality is crucial. This involves curating more accurate, diverse, and up-to-date datasets, ensuring they represent various contexts and cultures. By feeding AI systems with a stronger foundation of accurate information, the likelihood of hallucinations decreases.
- Training Tweaks: Advanced training techniques like cross-validation and more comprehensive datasets help address issues like overfitting and underfitting. Additionally, researchers are exploring ways to incorporate better contextual understanding into AI models. This allows the AI to grasp nuances more effectively, leading to fewer hallucinations.
- Algorithmic Advancements: One promising approach is Explainable AI (XAI). By understanding how an AI system reaches a conclusion, developers can pinpoint and correct the sources of hallucination. This transparency fosters trust and reliability in AI systems.
Real-World Consequences: Why Hallucination Matters
AI hallucination isn’t just a theoretical concern. It can have real-world consequences with serious implications:
- Healthcare Hazards: A study revealed that a popular chatbot provided inaccurate medical advice over 40% of the time. This highlights the importance of ensuring medical AI systems are accurate and reliable.
- Customer Service Stumbles: A chatbot providing incorrect information about refund policies can lead to customer dissatisfaction and financial losses.
- Legal Lapses: A lawyer using a chatbot to generate legal references accidentally included fabricated citations in a court brief. This underlines the necessity for human oversight in AI-generated legal advice to ensure accuracy and ethical conduct.
The Ethical Tightrope: Why We Need Responsible AI
The ethical implications of AI hallucination are significant. Misinformation from AI can have real-world consequences, endangering lives with incorrect medical advice and causing unjust outcomes with faulty legal advice. Regulatory bodies are addressing these concerns with proposals like the EU’s AI Act, which aims to establish guidelines for safe and ethical AI deployment. Transparency remains crucial, and XAI helps us understand how AI arrives at its outputs. This transparency is vital for identifying and correcting hallucinations, building trust in AI systems.
The Road Ahead: A Future Free from Hallucination?
AI chatbots are powerful tools, but their tendency for hallucination demands attention. By understanding the causes and implementing strategies to mitigate these errors, we can enhance the reliability and safety of AI systems. Continued advancements in data curation, model training, and explainable AI, coupled with essential human oversight, will ensure AI chatbots provide accurate and trustworthy information. This will ultimately lead to greater trust and utility for these powerful technologies, paving the way for a future where AI assistants offer truly intelligent and reliable support.