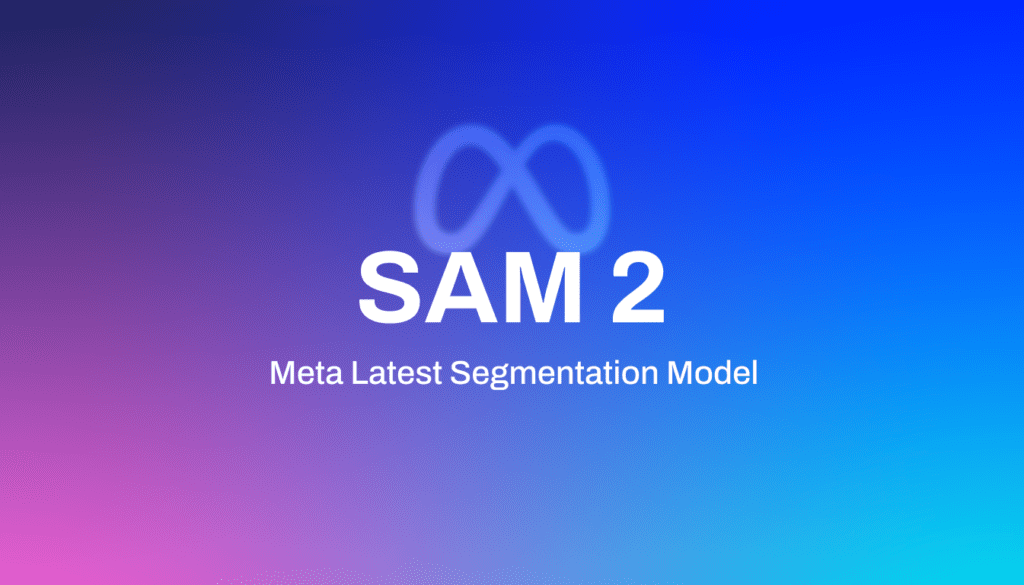
The realm of artificial intelligence has witnessed remarkable strides in text processing, with models like GPT revolutionizing industries. However, image and video understanding has lagged behind. Enter SAM 2, Meta’s groundbreaking open-source model, poised to redefine the landscape of computer vision.
From Static to Dynamic: The Evolution of Segmentation
Object segmentation, the art of isolating specific objects within an image or video, has traditionally been a complex task demanding vast amounts of data and computational resources. Meta’s inaugural Segment Anything Model (SAM) simplified this process by enabling users to segment objects with simple prompts.
SAM 2 takes this concept to new heights, extending its capabilities to video analysis. By treating each video frame as a connected sequence, SAM 2 can track objects across time, adapting to changes in lighting, occlusion, and movement.
The Power of Data and Architecture
At the heart of SAM 2’s prowess is its extensive training dataset, SA-V, comprising millions of video frames and corresponding object masks. This rich data, coupled with a refined model architecture, empowers SAM 2 to deliver unprecedented accuracy and speed.
The model’s ability to process information from previous frames is a game-changer, enabling it to maintain object consistency even in challenging scenarios. This advancement opens doors to a myriad of applications across industries.
Revolutionizing Industries with SAM 2
From healthcare to autonomous vehicles, SAM 2’s potential is vast. In healthcare, it can assist surgeons by providing real-time visualizations of anatomical structures, aiding in diagnosis and procedure planning. For autonomous vehicles, SAM 2 can enhance object detection and tracking, improving safety and navigation.
Beyond these sectors, SAM 2’s impact extends to entertainment, e-commerce, and environmental monitoring. By enabling precise object isolation and tracking, it can revolutionize video editing, product visualization, and wildlife conservation efforts.
Challenges and the Road Ahead
While SAM 2 is a significant leap forward, challenges persist. Tracking objects through complex scenes, handling occlusions, and maintaining accuracy in crowded environments remain areas for improvement. Additionally, optimizing the model for real-time performance on resource-constrained devices is crucial for widespread adoption.
Despite these limitations, SAM 2’s open-source nature fosters a collaborative environment for addressing these challenges. As researchers and developers build upon this foundation, we can expect even more groundbreaking advancements in computer vision.
Conclusion
SAM 2 marks a pivotal moment in the evolution of AI. By bridging the gap between image and video understanding, it empowers developers and researchers to explore new possibilities. As this technology matures, we can anticipate a future where AI seamlessly integrates into our lives, transforming industries and enhancing our experiences.