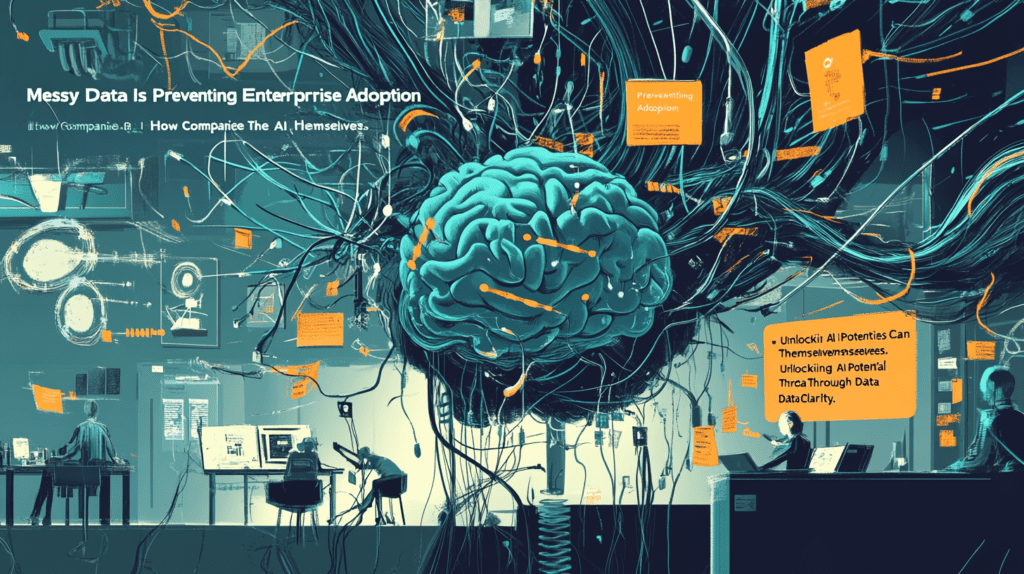
The healthcare industry’s cautious approach to AI, driven by the high stakes involved, is well understood. However, the enterprise software (SaaS) sector presents a curious paradox. While free from the same level of stringent regulation, it’s grappling with its own significant barrier to AI adoption: data chaos.
The Data Jungle
As businesses grow, so too does their data complexity. Initially, revenue data is often neatly contained within a single system. But as companies expand their product offerings, diversify sales channels, and adopt various pricing models, this pristine data landscape quickly transforms into a tangled jungle. The proliferation of SaaS tools, each with its own data silo, exacerbates the problem. Marketing departments, for instance, can easily accumulate two dozen or more data repositories.
This data fragmentation hinders visibility into the business’s inner workings. Manual data reconciliation becomes a time-consuming, error-prone task, often rendering insights obsolete by the time they’re available.
AI: A Tool, Not a Panacea
A common misconception is that AI can magically solve data chaos. While AI is a powerful tool, it’s not a magician. It demands clean, organized data to function effectively. Disparate, incompatible datasets, rife with inconsistencies and semantic variations, are an insurmountable challenge for AI. Even with data warehousing, extensive manual cleaning, labeling, and contextualization are prerequisites for deriving meaningful insights.
Furthermore, AI models are not infallible. They can produce hallucinations, which are especially problematic in finance where even minor inaccuracies can have far-reaching consequences. Human oversight remains crucial to ensure data accuracy and coherence.
Breaking Free from the Data Trap
To navigate this complex landscape, businesses must take proactive steps:
- Streamline the Tech Stack: Regularly assess the necessity of each SaaS tool. While some tools are indispensable, others might be contributing to the data tangle without providing commensurate value.
- Data-Centric Architecture: Prioritize metrics that align with the business’s overall goals. This approach helps in selecting software systems that support data coherence and analytical insights.
- Invest in Data Quality: Recognize that clean, organized data is the foundation for AI success. Allocate resources to data cleansing, standardization, and enrichment.
By investing in data quality and streamlining their tech stack, enterprises can pave the way for effective AI implementation. The journey may be challenging, but the rewards are substantial. Those who successfully untangle their data will gain a competitive edge through data-driven insights and automation.
In conclusion, while AI holds immense promise for enterprises, realizing its potential hinges on addressing the underlying data chaos. By taking a proactive approach to data management, businesses can unlock the true power of AI and drive innovation.