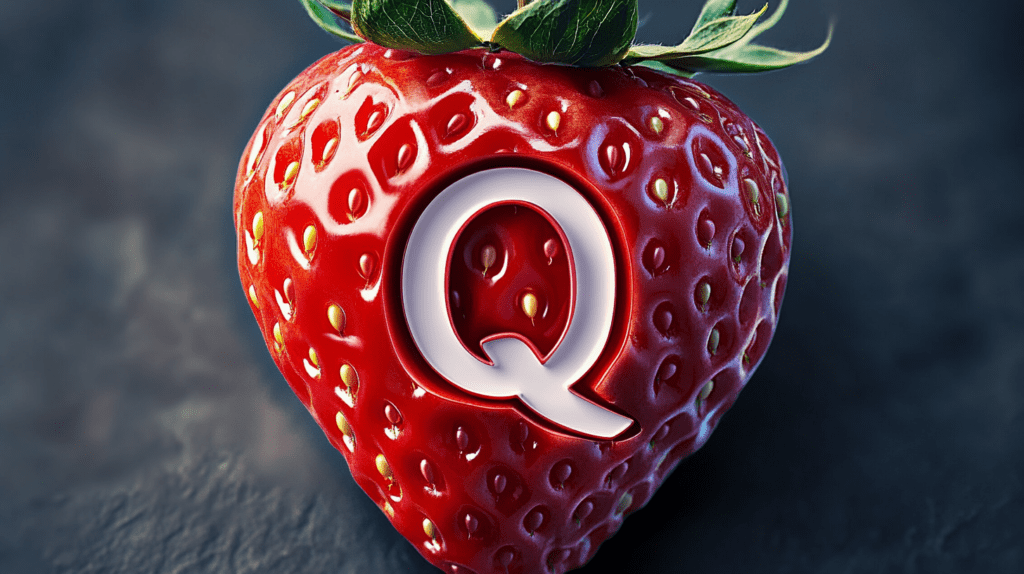
The Enigma of Q*
Q* remains one of the most tantalizing mysteries in the AI community, shrouded in secrecy and speculation. Despite being the subject of considerable intrigue, there is no official documentation or published research on it. OpenAI, the organization at the heart of this development, has been notably tight-lipped. Sam Altman, CEO of OpenAI, has only hinted at its existence, stating, “We are not ready to talk about that” during an interview.
In this blog, we will explore what little is known and delve into the plausible theories and assumptions surrounding Q*. Although much of the information is speculative, it is grounded in available research, expert opinions, and informed conjecture. As we navigate through these ideas, it’s crucial to approach them with an open mind and a healthy dose of skepticism. My goal is to make this complex topic accessible and engaging, ensuring that even those new to AI can grasp the core concepts.
The Genesis of Q*: A Breakthrough in AI?
Roughly six months ago, rumors began swirling about a groundbreaking development at OpenAI. Both Reuters and The Information reported on this potential breakthrough, which allegedly marks a significant leap toward achieving artificial general intelligence (AGI). This breakthrough, dubbed Q*, is believed to be a new algorithm or model that allows AI to self-learn and acquire logical, mathematical skills without external input—something current transformer architectures struggle to achieve.
The implications of this are enormous. AGI, by definition, is an AI that surpasses human capabilities in most economically valuable tasks. For this to become a reality, the AI must be capable of logical thinking, precise problem-solving, and expert-level knowledge across all domains. The advancements hinted at in Q* suggest that OpenAI may be closer to this goal than ever before, raising both excitement and concern within the AI community.
Unpacking the Potential of Q*
Q* is theorized to be a sophisticated algorithm designed to bridge the gap between current AI models and true human-like reasoning. Unlike today’s AI, which relies heavily on probability and statistical analysis, Q* may be capable of System 2 thinking—a term coined by Nobel laureate Daniel Kahneman. System 2 thinking involves deliberate, methodical, and logical reasoning, as opposed to the fast, intuitive, and automatic System 1 thinking.
Current AI models, like those built on transformer architectures, are excellent at tasks that require pattern recognition and prediction based on large datasets. However, they often fall short when faced with problems that require step-by-step logical reasoning. This is where Q* could potentially revolutionize AI. By enabling models to think in a more human-like, process-driven manner, Q* could eliminate the notorious problem of “hallucinations”—a term used to describe the false outputs generated by AI models when they lack sufficient information to make accurate predictions.
The Theories Behind Q*
While the exact workings of Q* remain unknown, several theories have emerged within the AI research community. Two prominent theories suggest that Q* might be an evolution of existing AI methodologies, combining elements of Q-learning and A* search algorithms.
- Q-Learning: This is a form of reinforcement learning where an AI agent learns to make decisions by interacting with its environment, without human intervention. The agent tries different actions and learns from the outcomes, gradually developing a strategy that maximizes its rewards. If Q* is built on Q-learning, it could signify a shift towards AI systems that can learn and adapt autonomously, without relying on vast amounts of pre-labeled data.
- A Search Algorithm*: A* is a well-known pathfinding and graph traversal algorithm used in many areas of computer science. It finds the shortest path between two points by evaluating potential paths based on a heuristic. When combined with Q-learning, the A* algorithm could enable AI to solve complex problems by systematically exploring and evaluating different possibilities, rather than relying solely on brute-force computation or random chance.
The Implications of Q* for the Future of AI
If Q* is indeed a combination of these advanced techniques, it could represent a monumental leap forward in AI capability. By integrating Q-learning’s ability to self-learn with A* search’s efficient problem-solving, Q* might enable AI to tackle tasks that were previously thought to be the exclusive domain of human intelligence. This could include everything from advanced scientific research to complex real-world decision-making processes.
However, the potential of Q* also comes with significant risks. The ability for an AI to learn and evolve autonomously, especially in the realm of logical reasoning and problem-solving, could lead to unforeseen consequences. The AI could become increasingly difficult to control, particularly if it begins to outperform humans in areas that require precise and logical thinking. This has already sparked concern among researchers and policymakers, who fear that we may be on the brink of creating AI systems that are too powerful for us to manage effectively.
Conclusion: A Future Shrouded in Mystery
As we await more concrete information about Q*, it’s clear that this development could mark a turning point in the quest for AGI. Whether Q* turns out to be the key to unlocking human-like reasoning in AI, or simply another step along the way, it has already ignited a wave of speculation and debate that will likely continue for years to come.
For now, all we can do is continue to explore the possibilities and prepare for the day when Q*—or something like it—finally emerges from the shadows. Until then, the mystery of Q* remains one of the most intriguing puzzles in the world of artificial intelligence.